Data extraction in evidence synthesis is labour-intensive, costly, and prone to errors. The use of large language models (LLMs) presents a promising approach for AI-assisted data extraction, potentially enhancing both efficiency and accuracy.
In this session, part of the Artificial Intelligence (AI) methods in evidence synthesis series, the presenter gave an overview of the current research landscape concerning data extraction using LLMs. The presenter also showed findings from a study within reviews (SWAR) that validated the workflow of employing an LLM for semi-automating data extraction within systematic reviews. Additionally, the webinar addressed current methodological challenges in evaluating LLMs for data extraction tasks.
This session was aimed at anyone conducting evidence synthesis. It was delivered in March 2025 and below you will find the videos from the webinar, together with the accompanying slides to download [PDF].
Part 1: Introduction and evaluations of LLMs for data extraction
Part 2: Practical tips for AI-assisted data extraction
Part 3: Questions and answers
Presenter Bio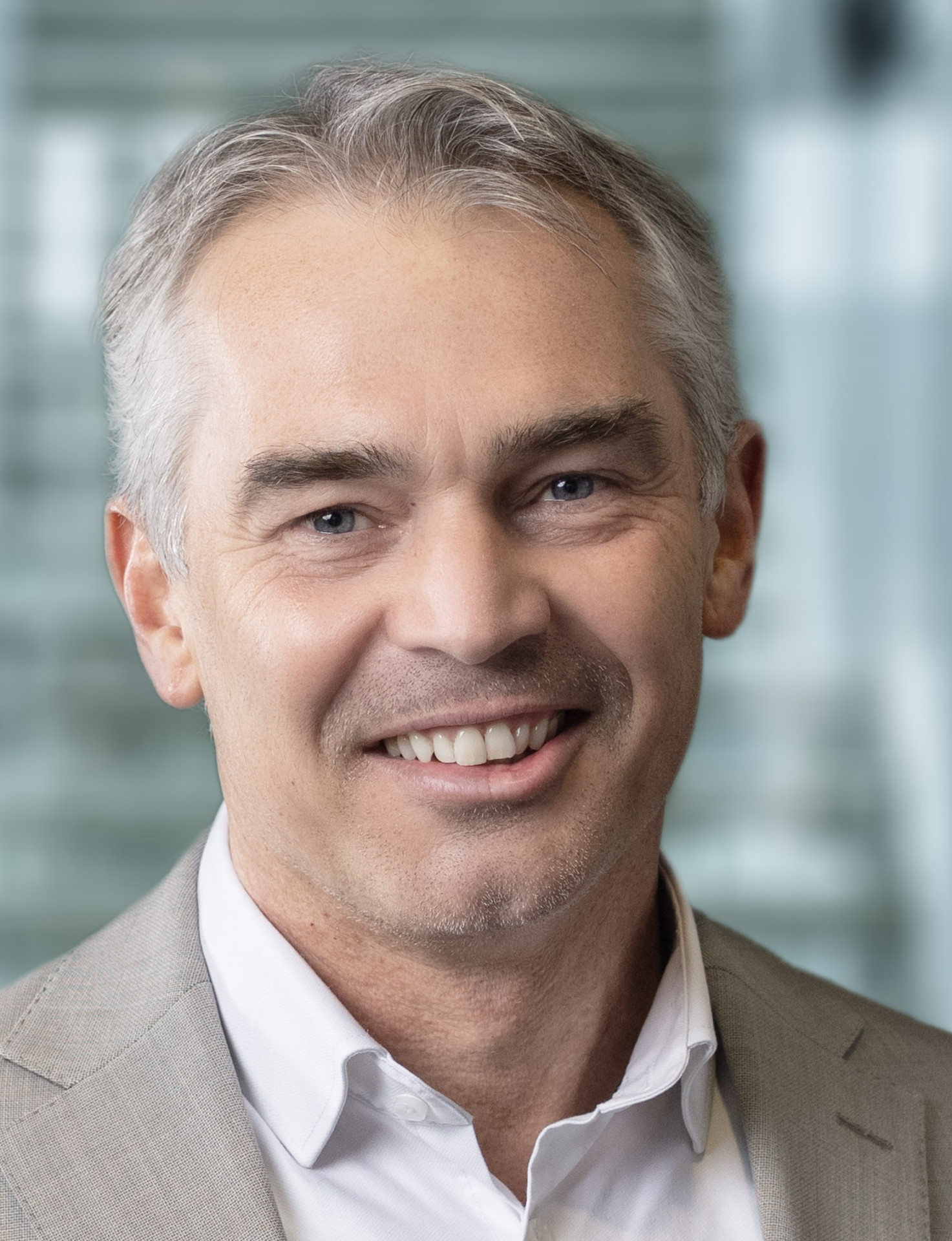
Gerald Gartlehner is a professor for evidence-based medicine and clinical epidemiology and the co-director of Cochrane Austria. He is also a co-convenor of the Cochrane Rapid Review Methods Groups. With over two decades of experience in conducting evidence synthesis, Gartlehner has recently focused on evaluating the performance of LLMs
for semi-automating various steps in the evidence synthesis processes.